Dr JunKyu Lee
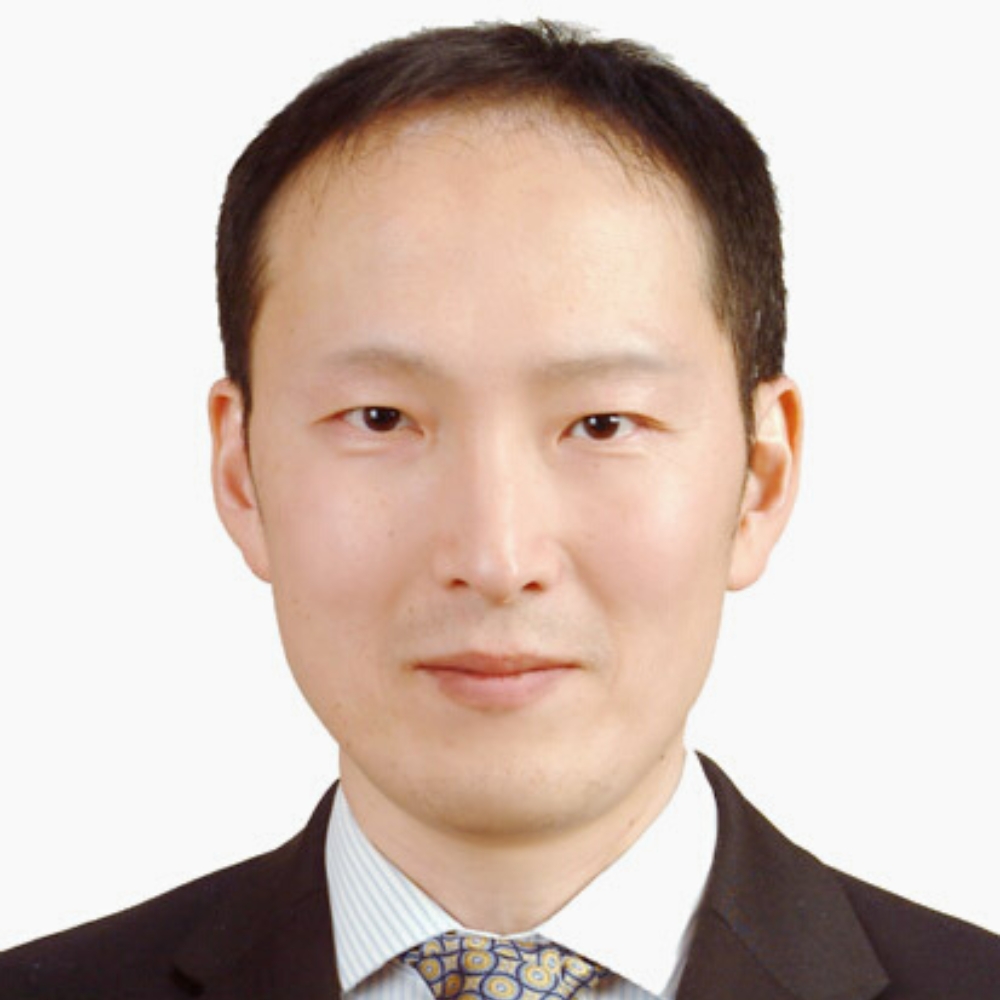
-
Email
j.lee@essex.ac.uk -
Location
Colchester Campus
Profile
Biography
JunKyu Lee received a Ph.D. degree in computer engineering from the University of Tennessee in 2012. Since then, he worked as a postdoctoral researcher, exploring energy-efficient machine learning at the Joint Institute for Computational Science (UT-ORNL) in the USA, at the University of Sydney in Australia, and at Queen's University Belfast in the UK, respectively. He is a research fellow in the Institute for Analytics and Data Science at the University of Essex in the UK. His research interests include linear algebra and machine learning, particularly exploring security-aware energy-efficient machine learning. He received a Marie Curie Fellowship in 2018.
Qualifications
-
PhD University of Tennessee at Knoxville,
Appointments
University of Essex
-
Research Fellow, Computer Science and Electronic Engineering, University of Essex (19/6/2023 - present)
Other academic
-
Research Fellow, Institute of Electronics Communications & Information Technology, Queen's University Belfast (5/6/2017 - 31/12/2022)
-
Research Associate, School of Electrical and Information Engineering, University of Sydney (5/1/2015 - 4/7/2016)
-
Post Doctoral Research Associate, Joint Institute for Computational Science, University of Tennessee - Oak Ridge National Laboratory (26/9/2012 - 31/12/2013)
Research and professional activities
Research interests
Energy-efficient AI, Trustworthy AI, Machine learning, tinyML, Federated learning, Kernel methods, Signal processing, FPGAs, Numerical linear algebra, Resource-efficient convolutional networks, Real-time object detection
Establishing the mathematical and computational foundation of energy-efficient machine learning, Accuracy and stability analysis of machine learning according to dynamically varying environments, and Incorporating numerical linear algebra properties into machine learning frameworks.
Teaching and supervision
Current teaching responsibilities
-
Web Development (CE154)
-
High Level Digital Design (CE339)
-
High Level Logic Design (CE869)
Current supervision
Publications
Publications (6)
Zhu, X., Zhang, H., Lee, J., Zhu, J., Pal, C., Saha, S., McDonald-Maier, KD. and Zhai, X., (2024). Fast, Scalable, Energy-Efficient Non-element-wise Matrix Multiplication on FPGA
Song, P., Lee, J. and Mukhanov, L., (2023). A case study on latency, bandwidth and energy efficiency of mobile 5G and YouTube Edge service in London. Why the 5G ecosystem and energy efficiency matter?
Park, J-I., Seong, S., Lee, J. and Hong, C-H., (2023). Vortex Feature Positioning: Bridging Tabular IIoT Data and Image-Based Deep Learning
Lee, J., Varghese, B. and Vandierendonck, H., (2022). ROMA: Run-Time Object Detection To Maximize Real-Time Accuracy
Lee, J., Mukhanov, L., Molahosseini, AS., Minhas, U., Hua, Y., Rincon, JMD., Dichev, K., Hong, C-H. and Vandierendonck, H., (2021). Resource-Efficient Deep Learning: A Survey on Model-, Arithmetic-, and Implementation-Level Techniques
Lee, J., Varghese, B., Woods, R. and Vandierendonck, H., (2021). TOD: Transprecise Object Detection to Maximise Real-Time Accuracy on the Edge
Journal articles (13)
Molahosseini, AS., Lee, J. and Vandierendonck, H., (2025). Software-Defined Number Formats for High-Speed Belief Propagation. IEEE Transactions on Emerging Topics in Computing, 1-14
Park, J-I., Seong, S., Lee, J. and Hong, C-H., (2025). Vortex Feature Positioning: Bridging tabular IIoT data and image-based deep learning. Internet of Things. 31, 101533-101533
Giménez, NL., Lee, J., Freitag, F. and Vandierendonck, H., (2024). The Effects of Weight Quantization on Online Federated Learning for the IoT: A Case Study. IEEE Access. 12, 5490-5502
Lee, J., Mukhanov, L., Molahosseini, AS., Minhas, U., Hua, Y., Martinez del Rincon, J., Dichev, K., Hong, C-H. and Vandierendonck, H., (2023). Resource-Efficient Convolutional Networks: A Survey on Model-, Arithmetic-, and Implementation-Level Techniques. ACM Computing Surveys. 55 (13s), 1-36
Minhas, UI., Lee, J., Mukhanov, L., Karakonstantis, G., Vandierendonck, H. and Woods, R., (2022). Increased Leverage of Transprecision Computing for Machine Vision Applications at the Edge. Journal of Signal Processing Systems. 94 (10), 1101-1118
Lee, J., Nikolopoulos, DS. and Vandierendonck, H., (2022). Mixed-Precision Kernel Recursive Least Squares. IEEE Transactions on Neural Networks and Learning Systems. 33 (3), 1284-1298
Lee, J. and Vandierendonck, H., (2021). Towards Lower Precision Adaptive Filters: Facts From Backward Error Analysis of RLS. IEEE Transactions on Signal Processing. 69, 3446-3458
Lee, J., Peterson, GD., Nikolopoulos, DS. and Vandierendonck, H., (2020). AIR: Iterative refinement acceleration using arbitrary dynamic precision. Parallel Computing. 97, 102663-102663
Lee, J., Vandierendonck, H., Arif, M., Peterson, GD. and Nikolopoulos, DS., (2018). Energy-Efficient Iterative Refinement Using Dynamic Precision. IEEE Journal on Emerging and Selected Topics in Circuits and Systems. 8 (4), 722-735
Fraser, NJ., Lee, J., Moss, DJM., Faraone, J., Tridgell, S., Jin, CT. and Leong, PHW., (2017). FPGA Implementations of Kernel Normalised Least Mean Squares Processors. ACM Transactions on Reconfigurable Technology and Systems. 10 (4), 1-20
Leong, PHW., Amano, H., Anderson, J., Bertels, K., Cardoso, JMP., Diessel, O., Gogniat, G., Hutton, M., Lee, J., Luk, W., Lysaght, P., Platzner, M., Prasanna, VK., Rissa, T., Silvano, C., So, HK-H. and Wang, Y., (2017). The First 25 Years of the FPL Conference. ACM Transactions on Reconfigurable Technology and Systems. 10 (2), 1-17
Lee, J., Peterson, GD., Harrison, RJ. and Hinde, RJ., (2010). Implementation of Hardware-Accelerated Scalable Parallel Random Number Generators. VLSI Design. 2010, 1-11
Lee, J., Bi, Y., Peterson, GD., Hinde, RJ. and Harrison, RJ., (2009). HASPRNG: Hardware Accelerated Scalable Parallel Random Number Generators. Computer Physics Communications. 180 (12), 2574-2581
Conferences (11)
Song, P., Lee, J., Abdelmoniem, AM. and Mukhanov, L., (2024). Do 5G Networks Achieve The Proclaimed Promises? An Empirical Study Using YouTube Edge Service
Lee, J., Varghese, B. and Vandierendonck, H., (2023). ROMA: Run-Time Object Detection To Maximize Real-Time Accuracy
Gimenez, NL., Freitag, F., Lee, J. and Vandierendonck, H., (2022). Comparison of Two Microcontroller Boards for On-Device Model Training in a Keyword Spotting Task
Lee, J., Varghese, B., Woods, R. and Vandierendonck, H., (2021). TOD: Transprecise Object Detection to Maximise Real-Time Accuracy on the Edge
Leong, PHW., Amano, H., Anderson, J., Bertels, K., Cardoso, JMP., Diessel, O., Gogniat, G., Hutton, M., Lee, J., Luk, W., Lysaght, P., Platzner, M., Prasanna, VK., Rissa, T., Silvano, C., So, H. and Yu Wang, (2015). Significant papers from the first 25 years of the FPL conference
Fraser, NJ., Moss, DJM., JunKyu Lee, Tridgell, S., Jin, CT. and Leong, PHW., (2015). A fully pipelined kernel normalised least mean squares processor for accelerated parameter optimisation
Lee, J. and Peterson, GD., (2012). The Role of Precision for Iterative Refinement
Liang, G., Lee, J. and Peterson, GD., (2012). ALU Architecture with Dynamic Precision Support
Lee, JK. and Peterson, GD., (2011). Iterative Refinement on FPGAs
(2010). Poster abstracts
JunKyu Lee, Peterson, GD., Harrison, RJ. and Hinde, RJ., (2008). Hardware accelerated Scalable Parallel Random Number Generators for Monte Carlo methods
Grants and funding
2024
PALLETS - Proactive AI-powered soLutions for Logistics Efficiency, Transparency and Safety
Innovate UK (formerly Technology Strategy Board)