People
Sirui Zhu
Assistant Lecturer
Department of Government
Postgraduate Research Student
Centre for Computational Finance and Economic Agents
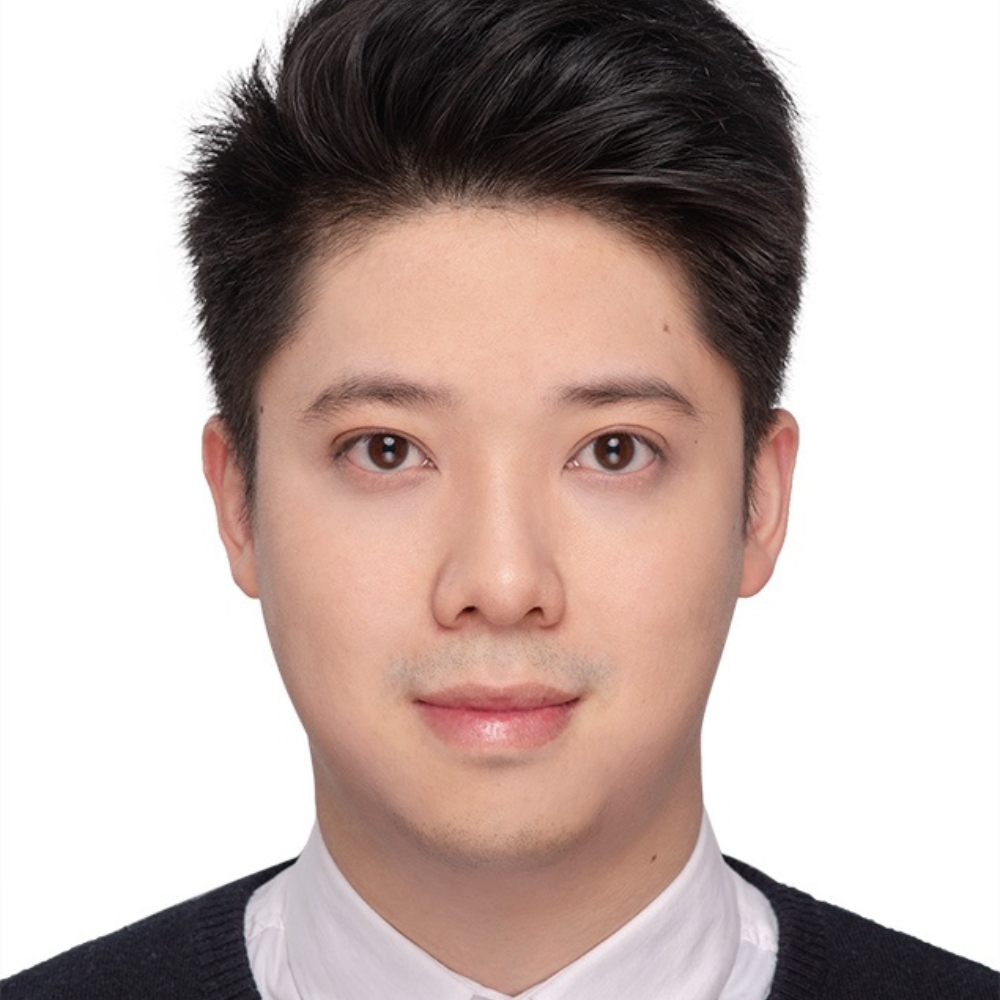
-
Email
sirui.zhu@essex.ac.uk -
Location
Colchester Campus